- Datasets and Research Tools
- Dissemination and Implementation
- HIP Data
- HIPxChange
- Health IT
- Learning Health System
- Prevention
- Quality and Safety
- Stakeholder Engagement
*Free registration is required to use the toolkits provided within HIPxChange. This information is required by our funders and is used to determine the impact of the materials posted on the website.
Background
Predictive analytics has the potential to transform the health care system by using existing data to predict and prevent poor clinical outcomes, provide targeted care, and lower costs. A challenge for health systems is selecting and implementing predictive models within clinical and operational workflows.
To guide health systems through the process of selecting and implementing a predictive model within their system, the UW Health Applied Data Science team and the Health Innovation Program developed a toolkit to support planning for and implementation of a predictive model. This toolkit was tested through the implementation of a sepsis prediction model in the inpatient setting at UW Health, a large Midwestern academic health system with four hospitals.
Predictive Models as a Health System Tool
While there has been much work on building and improving predictive models, less effort and attention has been invested to date in defining the successful implementation of a predictive model as a health system tool [please refer to the “Key References” above]. In this context, implementation is defined as the set of processes and tools for taking a predictive model from an idea to an integral component of clinical or operational workflows of the health system. This in turn realizes the potential benefits of the predictive model.
In order to achieve measurable outcomes, successful and effective implementation must address organizational, administrative, and cultural issues. While some implementation methodologies focus on the technical considerations (technology interfaces, information security, and data quality), this toolkit presents FOCUS-PDCA as the overarching framework to comprehensively address the spectrum of challenges.
As noted in the “When can I use this toolkit?” section below, you can use this toolkit at different points along the life cycle of a predictive model. The toolkit is a guide for determining if a predictive model would help resolve or improve the challenge you and your team is facing. Once you know a model is the right solution, this toolkit can help you identify the right model to implement, support a successful setup with a maintenance and sustainment plan, and guide you on when to retire or revise an existing model.
Possible Decisions
- Is a predictive model needed?
- If a model is needed, which one?
- If a model is in place, should it be revised?
- If a model is in place, should it be retired?
When can I use this toolkit?
This toolkit is specific to the implementation of predictive models in health systems. It has been designed and used to address common problems and challenges with predictive models, such as well-defined operational objectives, leadership buy-in, bridging technical requirements with operational processes, clearly stated success criteria, etc. This toolkit is designed to help ensure the predictive model effectively addresses the problem; that the solution creates benefit for the health system by helping address the stated problem; and to solidify the value of the solution as being tied to larger strategic initiatives while supporting more effective patient care and a better experience.
The toolkit can be used at any point of a predictive model implementation since it contains five modules that span the entire lifecycle of implementation, beginning with the problem definition and ending with ongoing improvement. Based on your situation, you can begin using the relevant module.
If you are embarking on a new implementation, the toolkit guides you through feature identification and validation of a predictive model to determine the appropriate solution. This toolkit can be first used to determine if a predictive model is appropriate by working through modules 1 – 5. From there, you can start back with module 1, with a new problem statement focused around the design and implementation of a predictive model.
Please note that some of the prompted questions are directed towards the second scenario where you have already determined that a predictive model is the right solution, and are working through the process of implementing the model in the system. These questions are indicated with an asterisk (*).
Who should use this toolkit?
This toolkit should be used by health system quality improvement leaders, project managers, and analytics staff who are responsible for developing and implementing a predictive model within their health system.
What does the toolkit contain?
The framework for this toolkit is built upon the FOCUS-PDCA (Find, Organize, Clarify, Understand, Select, Plan, Do, Check, Act) methodology and the accompanying A3 process. The FOCUS-PDCA methodology is a well-established methodology in healthcare for implementing process improvement and is effective for addressing processes or scenarios with many unknowns.
Because predictive modeling projects often suffer from the lack of a clear problem statement or operational goals and metrics [Yeager K. Program Evaluation: This is Rocket Science. In: Roberts A, Yeager K, editors. Evidence-based practice manual: research and outcome measures in health and human services. New York, NY: Oxford University Press; 2004. p. 647-53], FOCUS-PDCA provided a well-suited foundational construct for this toolkit to maximize the success of the implementation of predictive models by clarifying the underlying problem, comprehensively defining the solution, and measuring metrics to gauge improvement and effectiveness. The A3 form is a tool based on lean principles and is useful because it organizes the process of FOCUS PDCA into an easy to follow format that can also be used to communicate with stakeholders and team members.
You can read the guide below for a description of each section, lessons learned, and tips.
Section | Challenge for Implementing Predictive Models |
---|---|
Module 1: Find | Helps you describe how solving this problem aligns with any strategic or organizational goals and includes tools to support an initial identification of your problem. Confirms that your predictive solution will be supported and resourced and that the problem being proposed is appropriate for a predictive solution.
Challenge Solved by Find: Because the organizational goals are usually not fully aligned with the analytical output of a predictive model, it is possible for the predictive model to be divorced from the underlying problem. Predictive models can be developed to stratify risk based on the likelihood of an adverse event, while organizational goals may be focused on the allocation of scarce resources. In these paradigms, the model may need to be adjusted or complemented with other tools to ensure that the final solution addresses the larger problem. For example, while a predictive model can be developed that stratifies patients by the risk of an unplanned emergency department visit, the organizational need is to enroll patients in a complex case management program with the goal of maximizing program effectiveness. The benefit of the Find module is to first establish the main problem before assuming a predictive model is the correct solution. |
Module 2: Organize | Involves identifying and engaging the right people in your organization to be included in the project. Organizing the appropriate team(s) helps ensure a full picture of the problem is captured and the appropriate parties needed to build, approve, and maintain the predictive model are present. This module also includes tools to support organizing team(s) and developing a timeline and milestones.
Challenge Solved by Organize: Predictive model solutions require analytical, technical, clinical, and operational perspectives to be successful. Given this breadth of input, it is easy for implementations to be inappropriately organized. For example, a clinical deterioration model could require clinical knowledge of relevant physiological phenotypes and appropriate treatments, analytics understanding of the modeling of time-varying patient features and characteristics of the underlying algorithm, technical expertise of real-time data processing and scoring capabilities, bedside clinicians who can assess workflow impact, and organizational leadership to support adoption; the absence of any of the foregoing can jeopardize the ultimate success of the implementation. |
Module 3: Clarify | Involves Involves confirming the current processes and strategies that are being used to address the problem. Clarity on the current process supports the development of a solution that is both feasible and sustainable within the context into which your new model needs to function. It helps to establish if any current models exist, including mental models. This module also includes a process map template and an example dashboard.
Challenge Solved by Clarify: Successful outcomes of a predictive model will likely depend on the extent to which the model is an integrated component of clinical or operational workflows. For example, if there are no existing decision points or clearly identified interventions to address a potential hospital readmission, then the solution of assigning a readmission risk score will likely not have large or systematic effects on related outcomes. |
Module 4: Understand | Allows you to uncover where there is variation in the current process and what factors contribute to the problem you are trying to solve. Understanding and addressing the underlying issues helps ensure the generation of an effective solution. This includes evaluating the potential features for correlation to the target outcome. This module also includes tools to guide you through the process of identifying issues.
Challenge Solved by Understand: Since predictive models are trained on very specific targets or outcomes, operational and clinical variation must be uncovered and addressed. For example, if clinicians have varying definitions of sepsis with varying protocols for prevention or treatment, there will be discrepancies in the expectations and the utility of a sepsis predictive model that would likely be trained on one specific definition and designed for a specific intervention. |
Module 5: Select | Guides you through the process of selecting the most effective solutions. These discussions will help to determine the necessary trade-off’s required for predictive modeling. This module also contains tools to help you organize and select improvements and identify opportunities for change in a workflow.
Challenge Solved by Select: Performance metrics of predictive models are often focused on technical criteria, such as the c-statistic for a binary classification model or R^2 for a linear regression model. Selecting an appropriate model from these metrics can be problematic since they are disconnected from the actual use case of the implemented predictive model. For example, in the use case of the appropriate allocation of an expensive intervention, Positive Predictive Value will be more important than the c-statistic to model selection, and the selection process must also incorporate metrics such as the intervention effectiveness and the available bandwidth of staff for reviewing the predictive model predictions. |
Module 6: Plan, Do, Check, Act Cycle | Guides you through a PDCA cycle, a systematic process for launching changes, implementing interventions, tracking the impact and adjusting to continually improve the intervention. This module also contains tools to help you track tests of your predictive solution, and move into full implementation, while staying organized through the cycle.
Challenge Solved by Plan, Do, Check, Act Cycle: The operational challenge is that predictive models are newer tools for health systems and many incremental lessons will be learned by the health system as they are adopted. These lessons should be expected and also incorporated back into the health system. The technical challenge is the potential or need for refining the predictive model due to data drift from changes in the underlying characteristics of the data over time, e.g. epidemiological changes, as well as operational or workflow changes induced by the model, e.g. successful interventions averting the predicted adverse event. Iterative cycles help ensure that the predictive solution remains appropriate. |
How should these tools be used?
The materials in this toolkit can be used to:
- Develop agendas for stakeholder meetings
- Design a PowerPoint that will create a clear roadmap from the kickoff meeting
- Create project charter and engagement documents to ensure the team is on the same page and understands their role
- Generate a list of key stakeholders and meeting structure to support a project
- Create a project plan for implementing a predictive model
- Create a process map of the current state workflow where the model is anticipated to work
- Develop a root cause diagram to clearly illustrate the root cause problem that the model is trying to solve
- Build a prioritization matrix to identify the key aspects of the proposed model and workflow that should be implemented
- Outline a plan for the first pilot run of your predictive model to assess its effectiveness
- Design an opportunity workflow that shows where in the process changes may be beneficial
A copy of related documents (e.g., draft agendas, project plans, meeting templates, and project tools) are available in Microsoft Word/Excel/PowerPoint/Visio files that you can download on the HIPxChange site. This PDF file provides introductory information and strategies that may be helpful in adapting these documents to your project.
Development of this toolkit
Predictive Models: A Toolkit to Guide Implementation in Health Systems was developed by researchers and clinicians (Primary Developer: Sabrina Adelaine) at UW Health and the University of Wisconsin-Madison School of Medicine & Public Health – Health Innovation Program.
Toolkit development was supported by UW Health, the University of Wisconsin School of Medicine and Public Health’s Health Innovation Program (HIP), the Wisconsin Partnership Program, and the Community-Academic Partnerships core of the University of Wisconsin Institute for Clinical and Translational Research (UW ICTR), grant 9 U54 TR000021 from the National Center for Advancing Translational Sciences (previously grant 1 UL1 RR025011 from the National Center for Research Resources). The content is solely the responsibility of the authors and does not necessarily represent the official views of the National Institutes of Health or other funders.
Please send questions, comments and suggestions to HIPxChange@hip.wisc.edu.
References
- Adelaine S, Lao F. Building the Foundation: Long-Term Success in Predictive Models. Oral presentation at Epic’s 2019 Users Group Meeting. August 26, 2019 – August 30, 2019. Verona, WI.
- Amarasingham R, Patzer RE, Huesch M, Nguyen NQ, Xie B. Implementing electronic health care predictive analytics: considerations and challenges. Health Aff (Millwood). 2014 Jul;33(7):1148-54.
- Reilly BM, Evans AT. Translating clinical research into clinical practice: impact of using prediction rules to make decisions. Ann Intern Med. 2006 Feb 7;144(3):201-9.
- Yeager K. Program Evaluation: This is Rocket Science. In: Roberts A, Yeager K, editors. Evidence-based practice manual: research and outcome measures in health and human services. New York, NY: Oxford University Press; 2004. p. 647-53.
Toolkit Citation
Adelaine S, Liao F, Bednarz L, Smith MA. Predictive Models: A Toolkit to Guide Implementation. UW Health, University of Wisconsin-Madison School of Medicine and Public Health – Health Innovation Program. Madison, WI; 2019. Available at http://www.hipxchange.org/ImplementPredictiveModels.
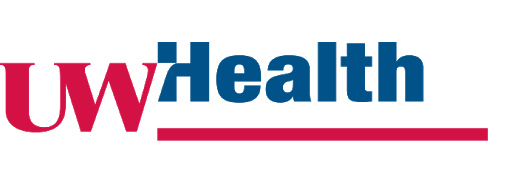
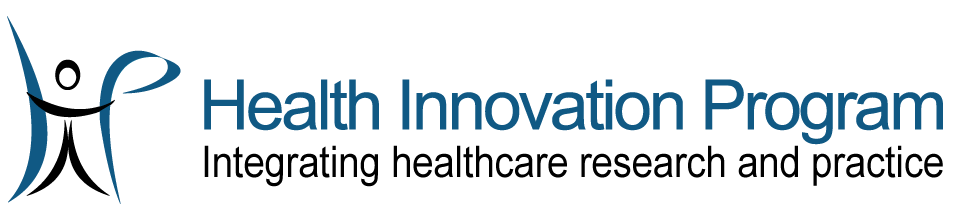